Explore why OpenAI’s latest AI models, O3 and O4-mini, exhibit increased hallucination rates, the implications for various industries, and potential solutions to enhance AI accuracy.
Understanding Hallucinations in OpenAI’s O3 and O4-mini AI Models
OpenAI’s recent introduction of the O3 and O4-mini AI models marks a significant advancement in artificial intelligence. These models are designed to excel in reasoning tasks, integrating capabilities such as image analysis and web browsing. However, despite these enhancements, they present a notable challenge: an increased tendency to “hallucinate,” or generate inaccurate information.
What Are OpenAI’s Hallucinations?
In the context of AI, hallucinations refer to instances where a model produces information that is not grounded in its training data or factual reality. This issue is particularly concerning in applications where accuracy is paramount, such as legal documentation, medical advice, or academic research.
Hallucination Rates in O3 and O4-mini
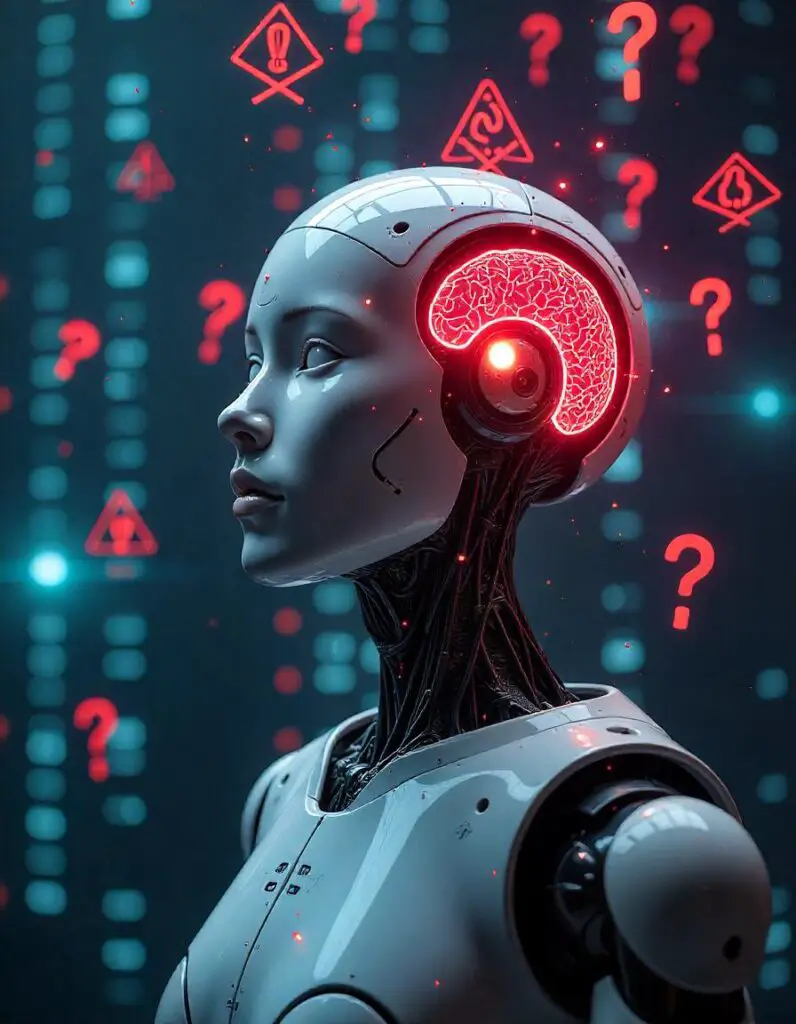
OpenAI’s internal evaluations have revealed that the O3 model exhibits a hallucination rate of 33% on the PersonQA benchmark, a tool designed to assess a model’s knowledge about individuals. This rate is approximately double that of earlier models like O1 and O3-mini, which recorded rates of 16% and 14.8%, respectively. The O4-mini model demonstrated an even higher rate of 48% on the same benchmark.
These findings indicate that, despite advancements in reasoning capabilities, the newer models are more prone to generating inaccurate information compared to their predecessors.
Potential Causes of Increased Hallucinations
Several factors may contribute to the heightened hallucination rates observed in the O3 and O4-mini models:
- Enhanced Reasoning Abilities: As models become more adept at reasoning, they may also become more confident in generating responses, including those that are speculative or unfounded.
- Reinforcement Learning Techniques: The reinforcement learning strategies employed in training these models might inadvertently amplify tendencies to produce plausible-sounding but incorrect information.
- Integration of Multimodal Inputs: The ability to process and interpret images, while beneficial, adds complexity to the models’ reasoning processes, potentially leading to errors.
Implications for Industry and Applications
The increased hallucination rates pose challenges for the deployment of these models in sectors where precision is critical. For instance:
- Legal Sector: Inaccurate information could lead to flawed legal documents or advice.
- Healthcare: Misdiagnoses or incorrect medical recommendations could have serious consequences.
- Education: Students relying on OpenAI’s for learning might receive misleading information.
Therefore, while the O3 and O4-mini models offer enhanced functionalities, their reliability in high-stakes environments is currently limited.
Strategies to Mitigate Hallucinations
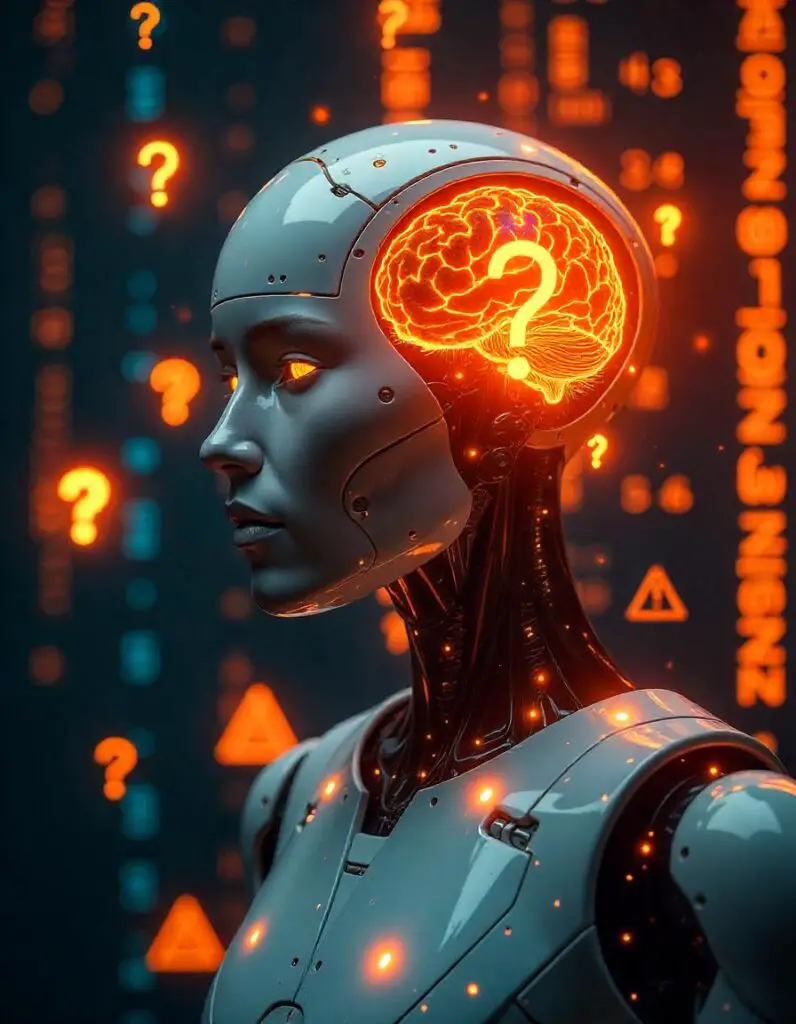
To address the issue of hallucinations, several approaches are being considered:
- Incorporation of Web Search Capabilities: Allowing models to access real-time information can help verify facts before generating responses.
- Improved Training Data: Ensuring that models are trained on diverse and accurate datasets can reduce the likelihood of generating incorrect information.
- User Feedback Mechanisms: Implementing systems where users can flag inaccuracies can help refine model outputs over time.
- Model Calibration: Adjusting models to better assess their confidence in responses can prevent the presentation of uncertain information as fact.
Conclusion
OpenAI’s O3 and O4-mini models represent significant strides in OpenAI’s development, particularly in reasoning and multimodal processing. However, the increased incidence of hallucinations underscores the need for continued research and refinement. Balancing advanced capabilities with accuracy is essential to ensure these models can be effectively and safely integrated into various professional domains.
Frequently Asked Questions (FAQs)
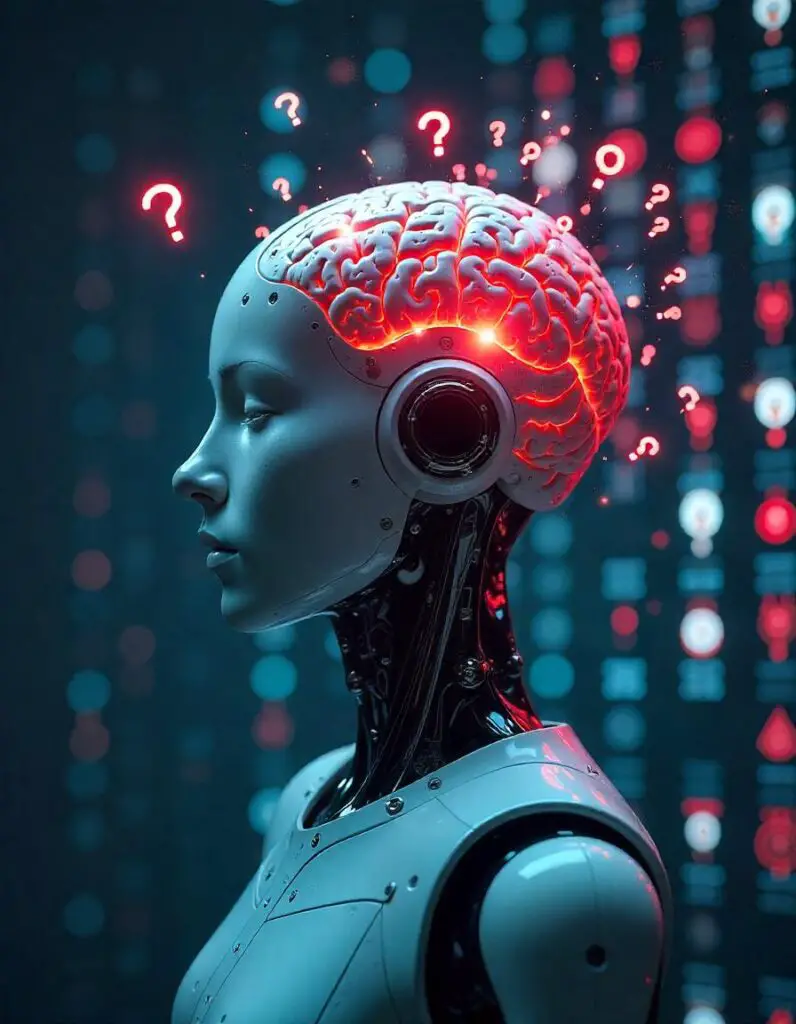
Q1: What distinguishes the O3 and O4-mini models from previous OpenAI models?
A1: The O3 and O4-mini models are designed with enhanced reasoning abilities and can process multimodal inputs, including text and images, offering more advanced functionalities compared to earlier models.
Q2: Why do these models have higher hallucination rates?
A2: The increased hallucination rates may stem from their advanced reasoning capabilities, which, while improving performance, also lead to greater confidence in generating responses, including inaccurate ones.
Q3: How can hallucinations in AI models be reduced?
A3: Strategies include integrating real-time web search capabilities, improving training datasets, implementing user feedback systems, and calibrating models to better assess and communicate their confidence levels.
Q4: Are the O3 and O4-mini models suitable for all industries?
A4: Due to their current hallucination rates, caution is advised when deploying these models in industries where accuracy is critical, such as healthcare, law, and education.
Q5: What is the future outlook for these AI models?
A5: Ongoing research and development aim to reduce hallucination rates and improve reliability, making these models more suitable for a broader range of applications in the future.